旋转框目标检测mmrotate v1.0.0rc1 之RTMDet训练DOTA的官方问题解析整理(四)
1、Batchsize和学习率问题
1、关于rotated_rtmdet_l-coco_pretrain-3x-dota_ms.py配置文件的batchsize和学习率设置
问题:
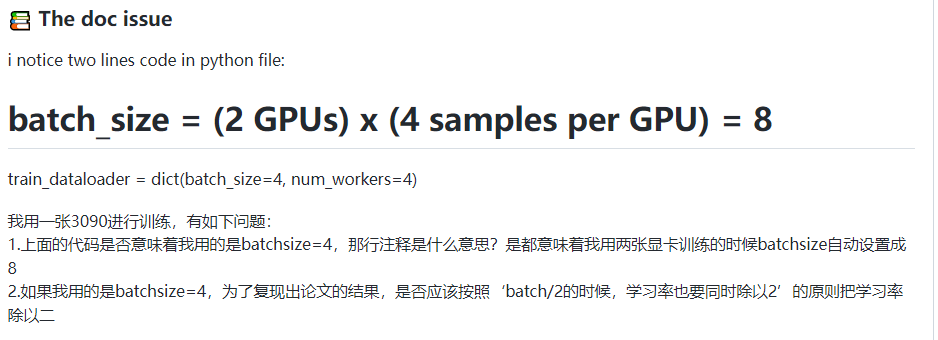
回答:

2、不同batchsize下s2anet的mAP #59
问题:
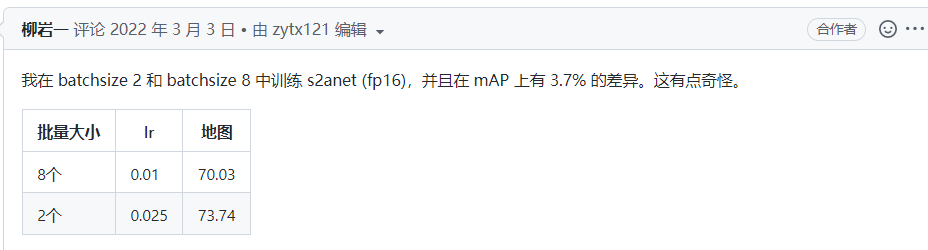
回答:
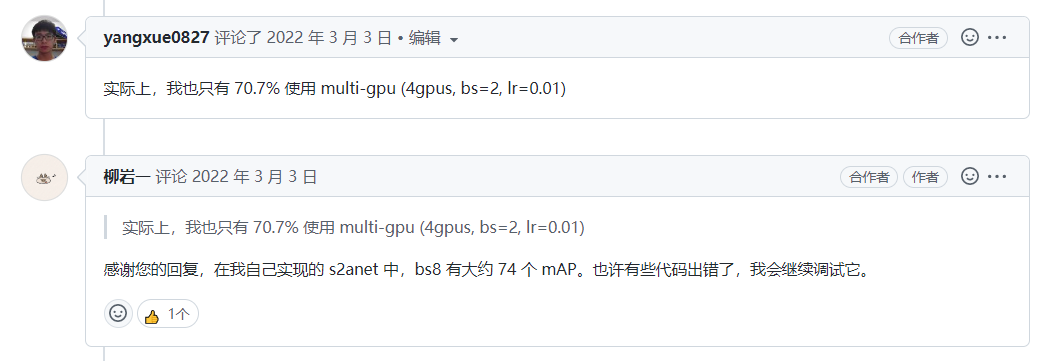
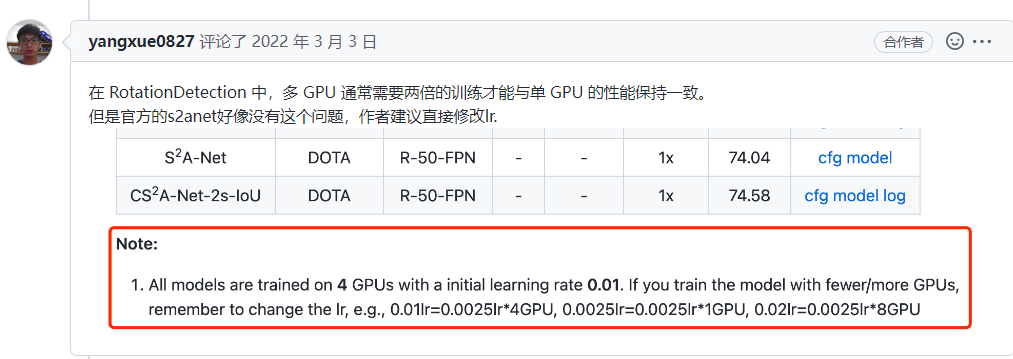
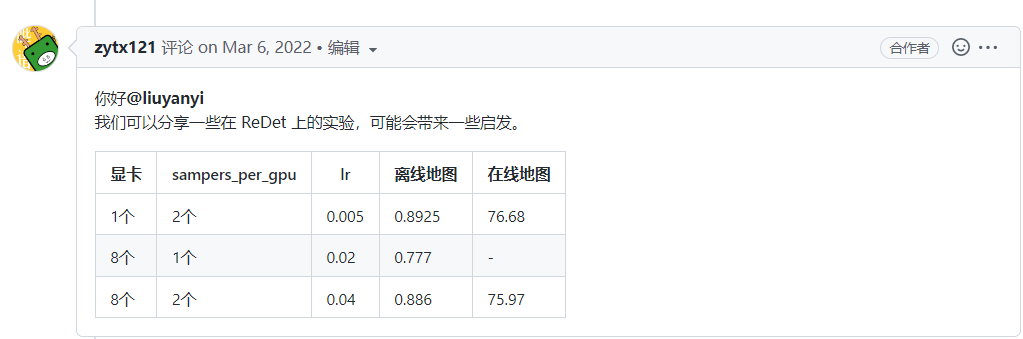
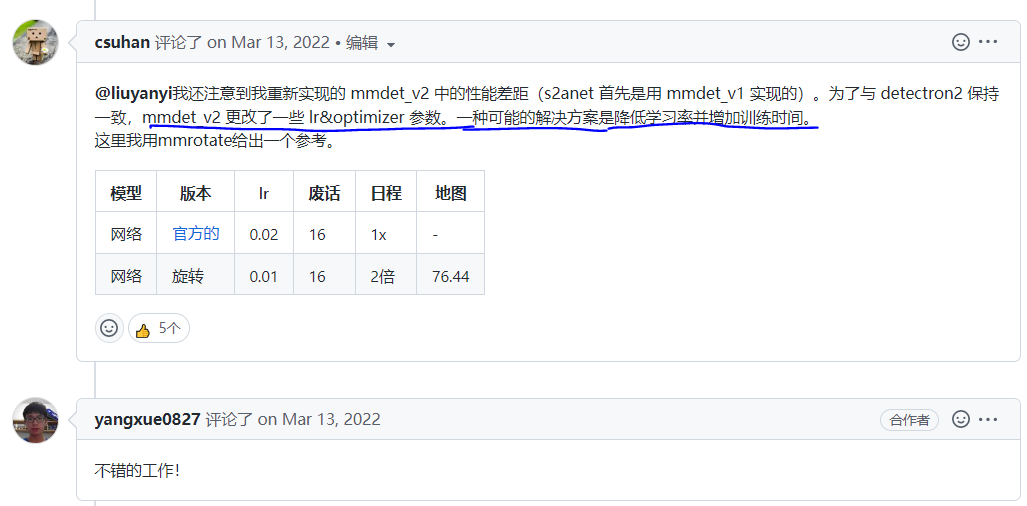
3、关于lr和batchsize的问题 #645
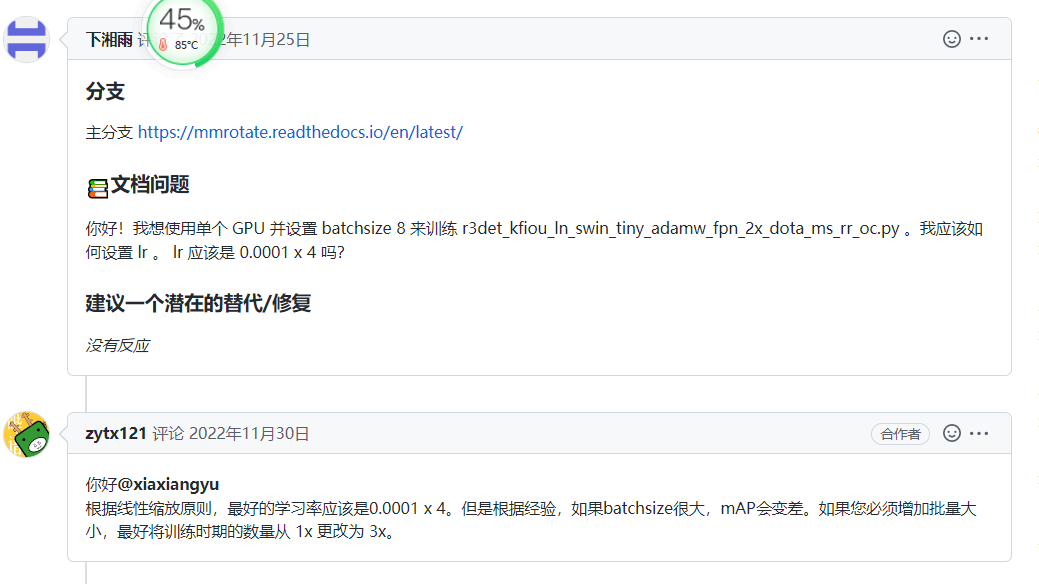
2、如何进行多尺度测试? #201
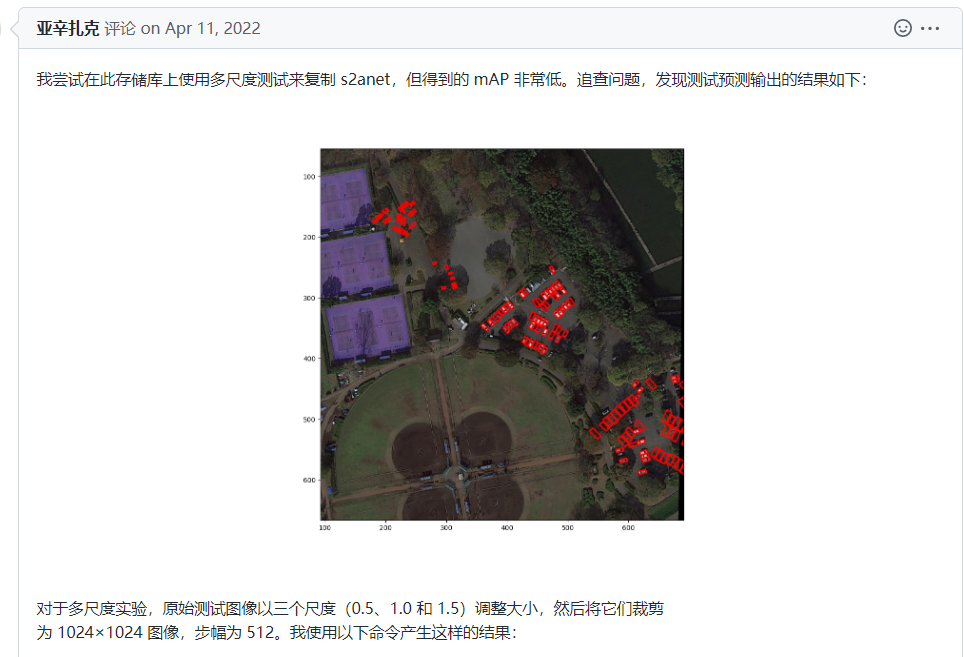
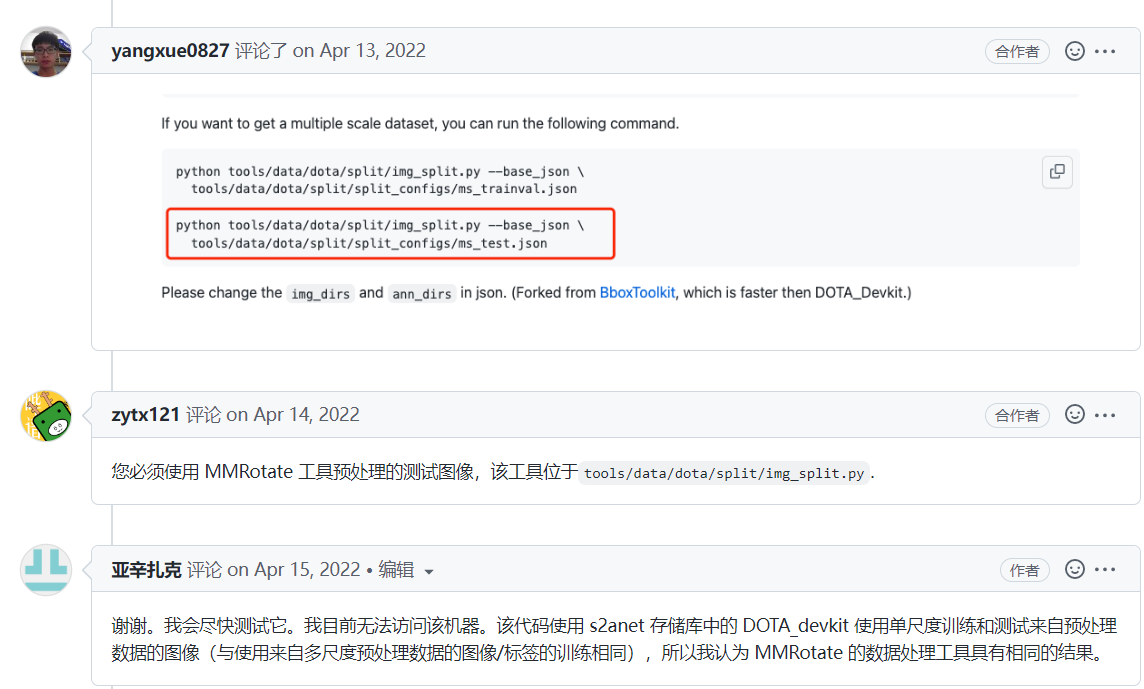
3、为什么相同的物体分类分数相差很大? #455
问题:
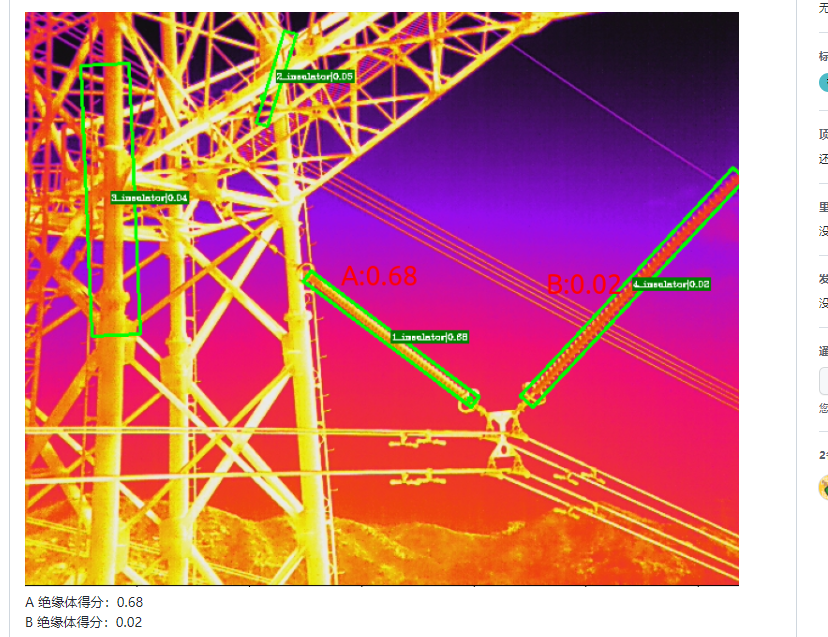
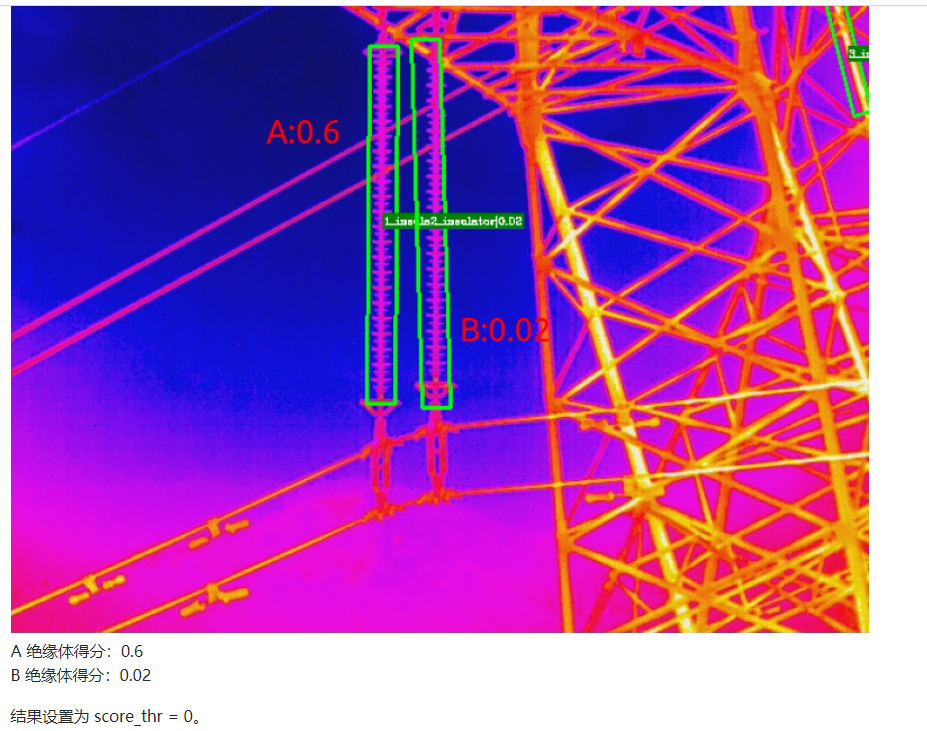

回答:
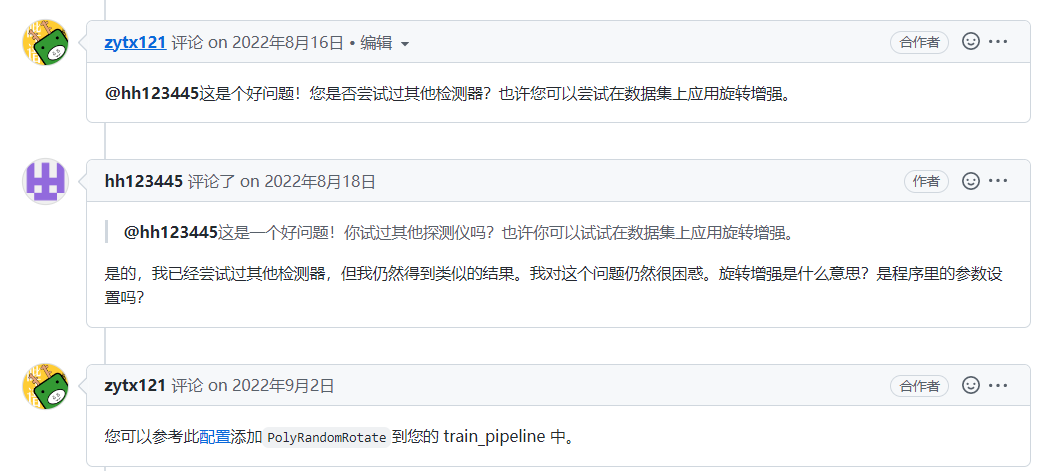
_base_ = ['./roi_trans_r50_fpn_1x_dota_le90.py']
data_root = 'datasets/split_ms_dotav1/'
angle_version = 'le90'
img_norm_cfg = dict(
mean=[123.675, 116.28, 103.53], std=[58.395, 57.12, 57.375], to_rgb=True)
train_pipeline = [
dict(type='LoadImageFromFile'),
dict(type='LoadAnnotations', with_bbox=True),
dict(type='RResize', img_scale=(1024, 1024)),
dict(
type='RRandomFlip',
flip_ratio=[0.25, 0.25, 0.25],
direction=['horizontal', 'vertical', 'diagonal'],
version=angle_version),
dict(
type='PolyRandomRotate',
rotate_ratio=0.5,
angles_range=180,
auto_bound=False,
rect_classes=[9, 11],
version=angle_version),
dict(type='Normalize', **img_norm_cfg),
dict(type='Pad', size_divisor=32),
dict(type='DefaultFormatBundle'),
dict(type='Collect', keys=['img', 'gt_bboxes', 'gt_labels'])
]
data = dict(
train=dict(
pipeline=train_pipeline,
ann_file=data_root + 'trainval/annfiles/',
img_prefix=data_root + 'trainval/images/'),
val=dict(
ann_file=data_root + 'trainval/annfiles/',
img_prefix=data_root + 'trainval/images/'),
test=dict(
ann_file=data_root + 'test/images/',
img_prefix=data_root + 'test/images/'))
model = dict(train_cfg=dict(rpn=dict(assigner=dict(gpu_assign_thr=200))))
4、检测细长物体的困难 #384
问题:
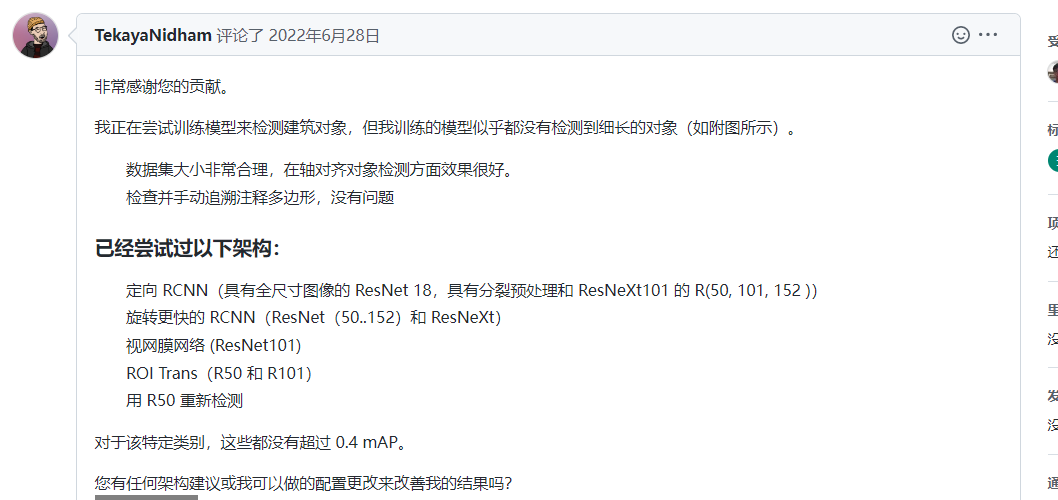
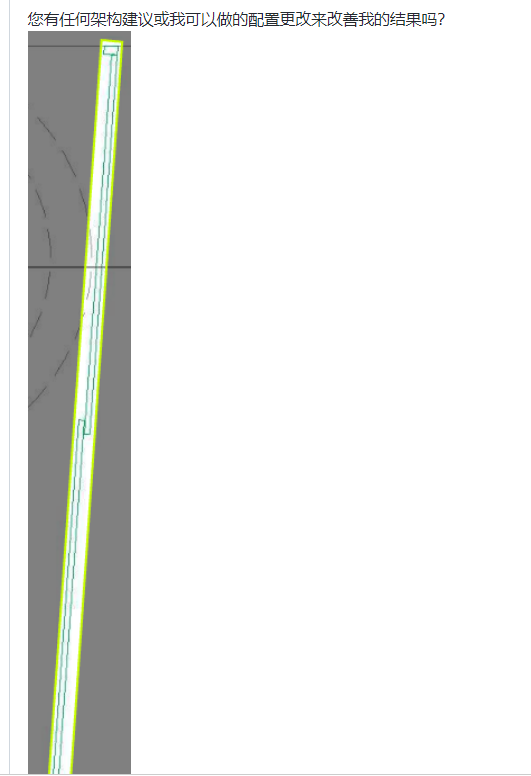
回答:
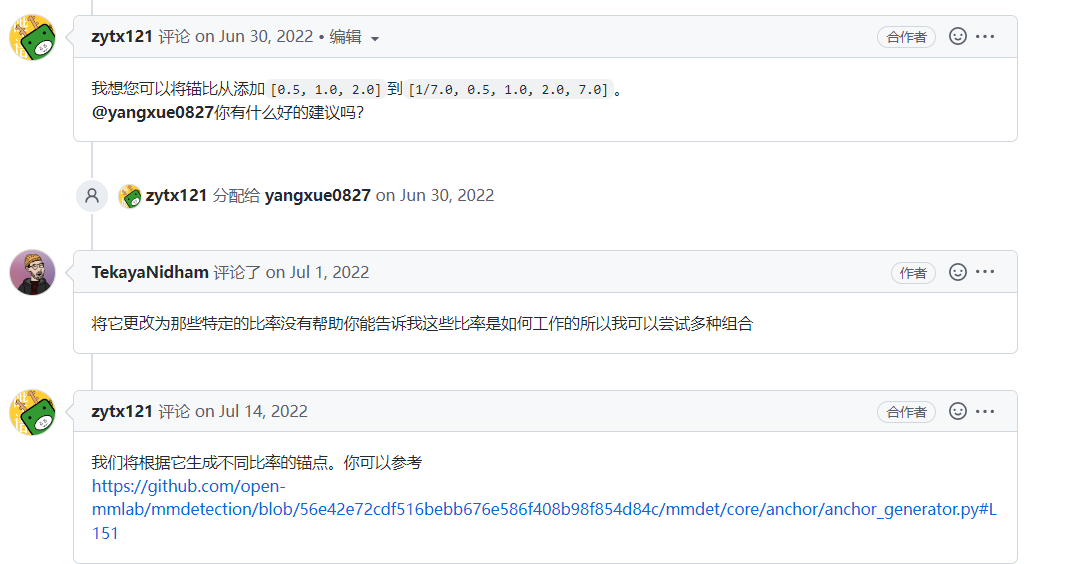
def gen_single_level_base_anchors(self,
base_size,
scales,
ratios,
center=None):
"""Generate base anchors of a single level.
Args:
base_size (int | float): Basic size of an anchor.
scales (torch.Tensor): Scales of the anchor.
ratios (torch.Tensor): The ratio between between the height
and width of anchors in a single level.
center (tuple[float], optional): The center of the base anchor
related to a single feature grid. Defaults to None.
Returns:
torch.Tensor: Anchors in a single-level feature maps.
"""
w = base_size
h = base_size
if center is None:
x_center = self.center_offset * w
y_center = self.center_offset * h
else:
x_center, y_center = center
h_ratios = torch.sqrt(ratios)
w_ratios = 1 / h_ratios
if self.scale_major:
ws = (w * w_ratios[:, None] * scales[None, :]).view(-1)
hs = (h * h_ratios[:, None] * scales[None, :]).view(-1)
else:
ws = (w * scales[:, None] * w_ratios[None, :]).view(-1)
hs = (h * scales[:, None] * h_ratios[None, :]).view(-1)
# use float anchor and the anchor's center is aligned with the
# pixel center
base_anchors = [
x_center - 0.5 * ws, y_center - 0.5 * hs, x_center + 0.5 * ws,
y_center + 0.5 * hs
]
base_anchors = torch.stack(base_anchors, dim=-1)
return base_anchors
5、如何训练宽高比大的物体 #285
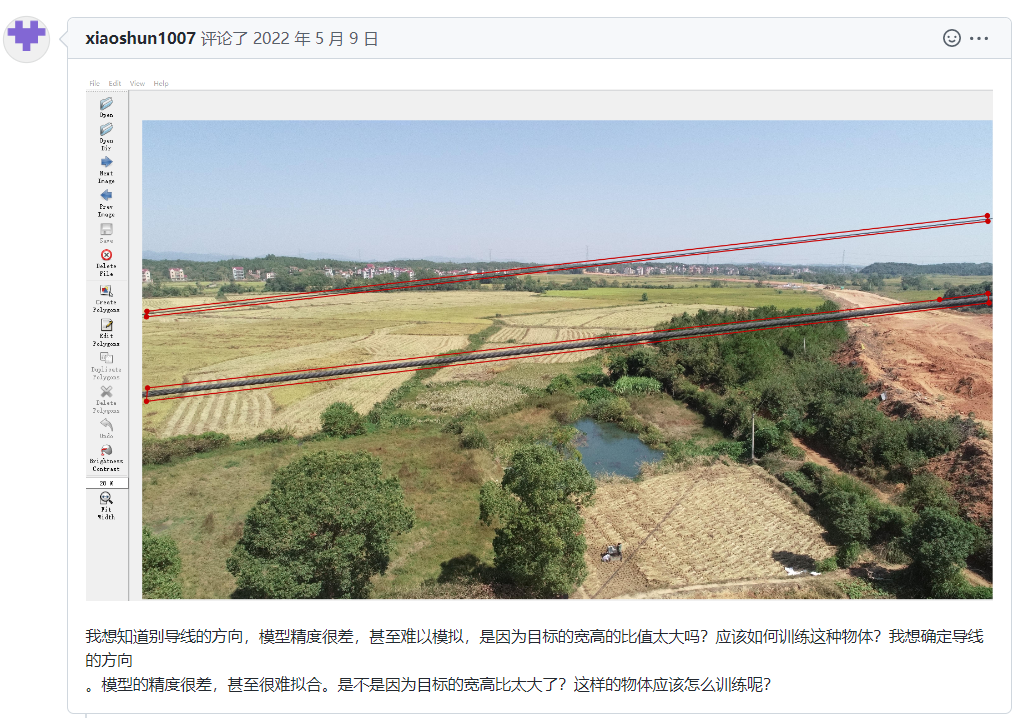
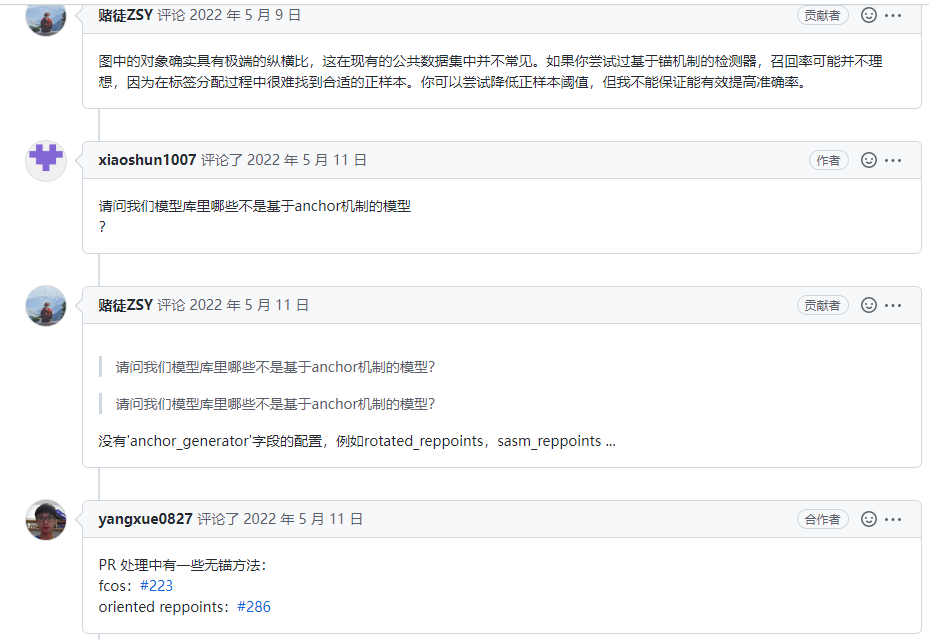
6、请问这个项目中通道的ms+rr和论文中常说的多维度测试和多维度训练有什么区别
问题:
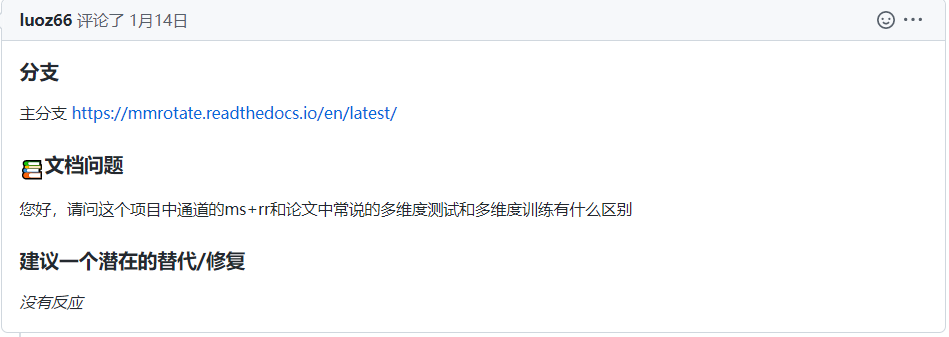
回答:
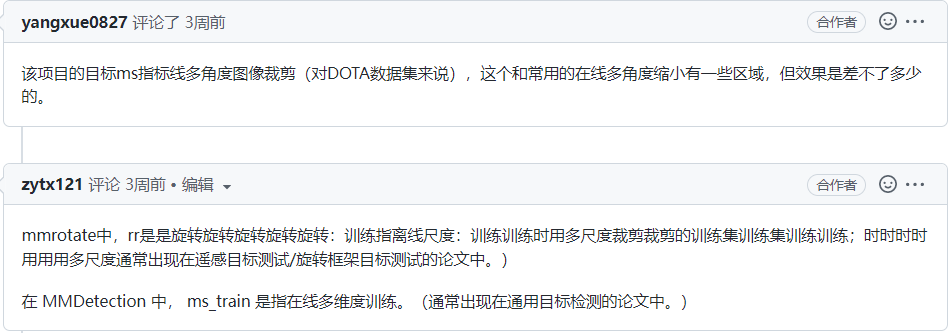
7、如何在mmrotate中绘制特征图
问题:
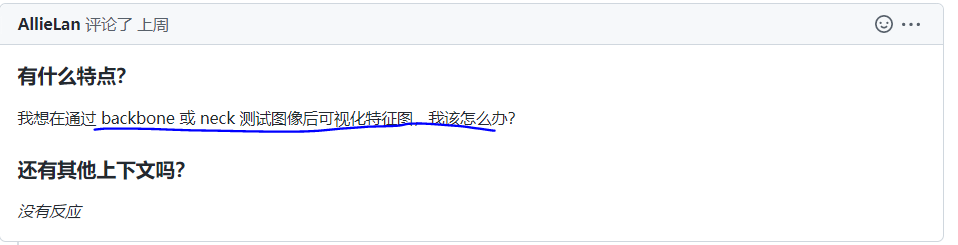
回答:
你好@AllieLan,您可以尝试使用https://github.com/open-mmlab/mmyolo/blob/main/demo/featmap_vis_demo.py
8、oriented reppoints 支持 filter_empty_gt=False 的训练
问题:
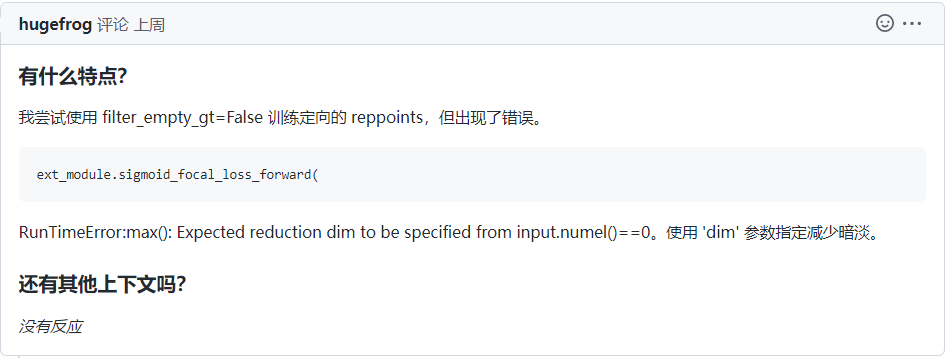
回答:

9、[1.x] RTMDet-R (tiny) 内存不足的 CUDA,具有 24GB VRAM 和 batch_size=1
问题:
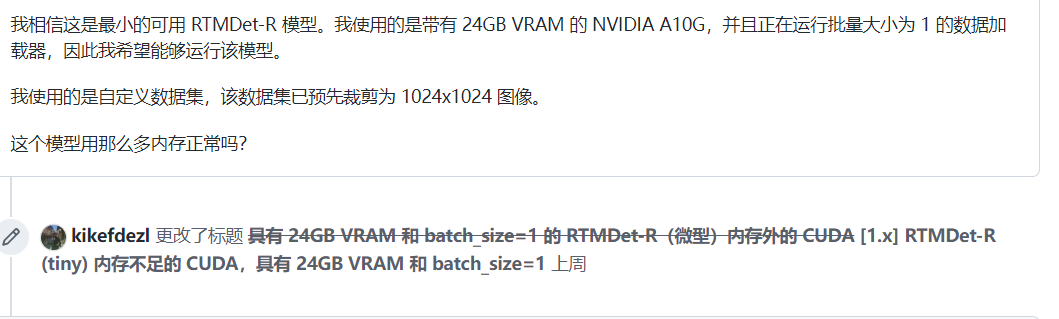
回答:
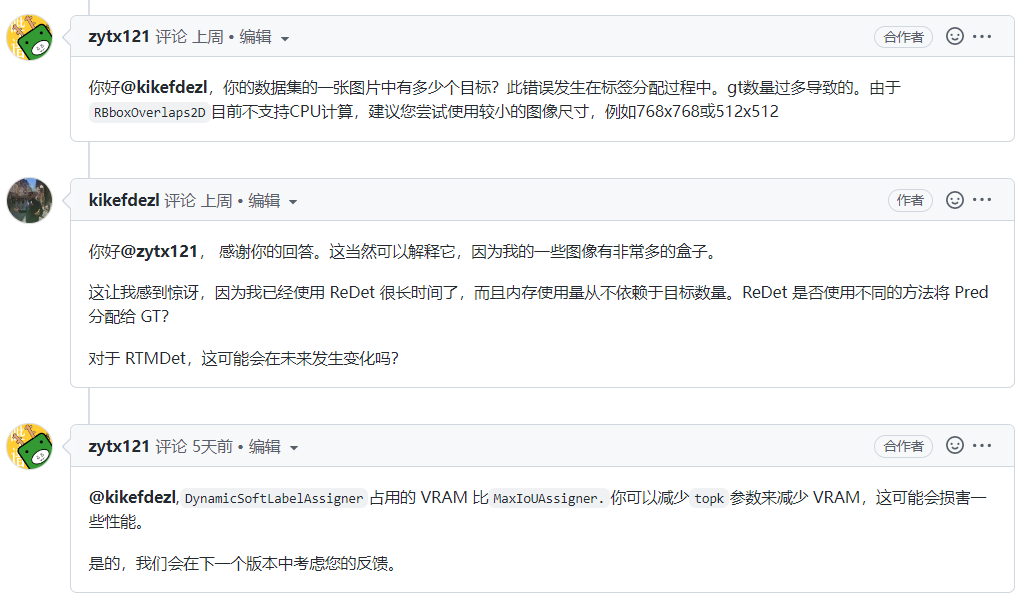
10、如何在自己的数据集上测试大场景图片?
问题:
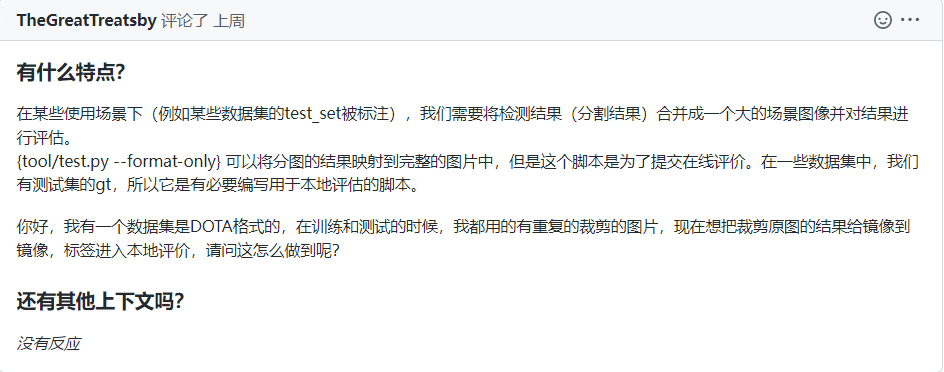
回答:
你好@TheGreatTreatsby, 你可以试试https://github.com/CAPTAIN-WHU/DOTA_devkit
11、使用 DOTA V1.0 数据集时 CFA 重新分配过程中的张量不匹配错误
问题:

回答:
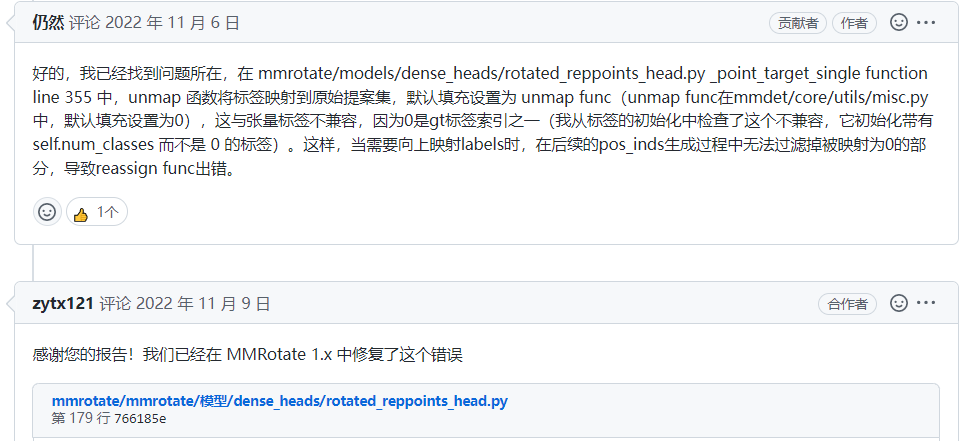
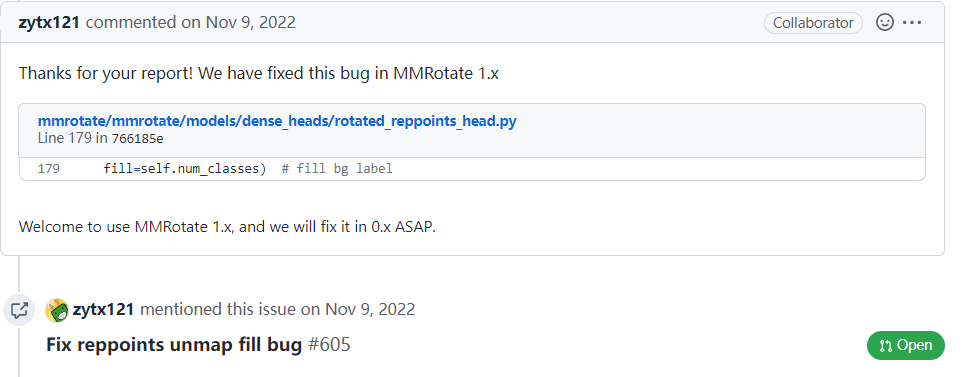
12、如何改变旋转框的定义范围(如何更改旋转框的定义范围)
问题:

回答:
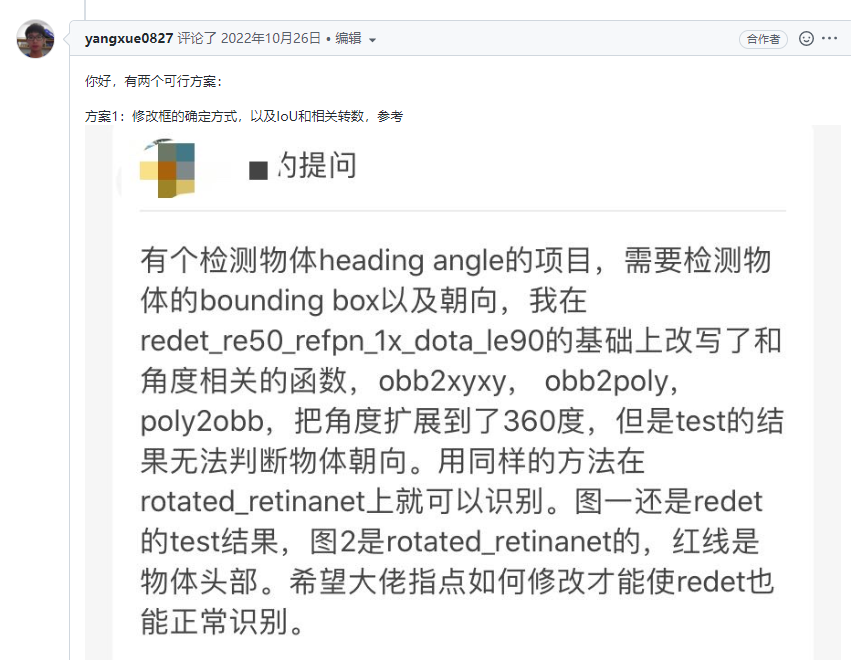
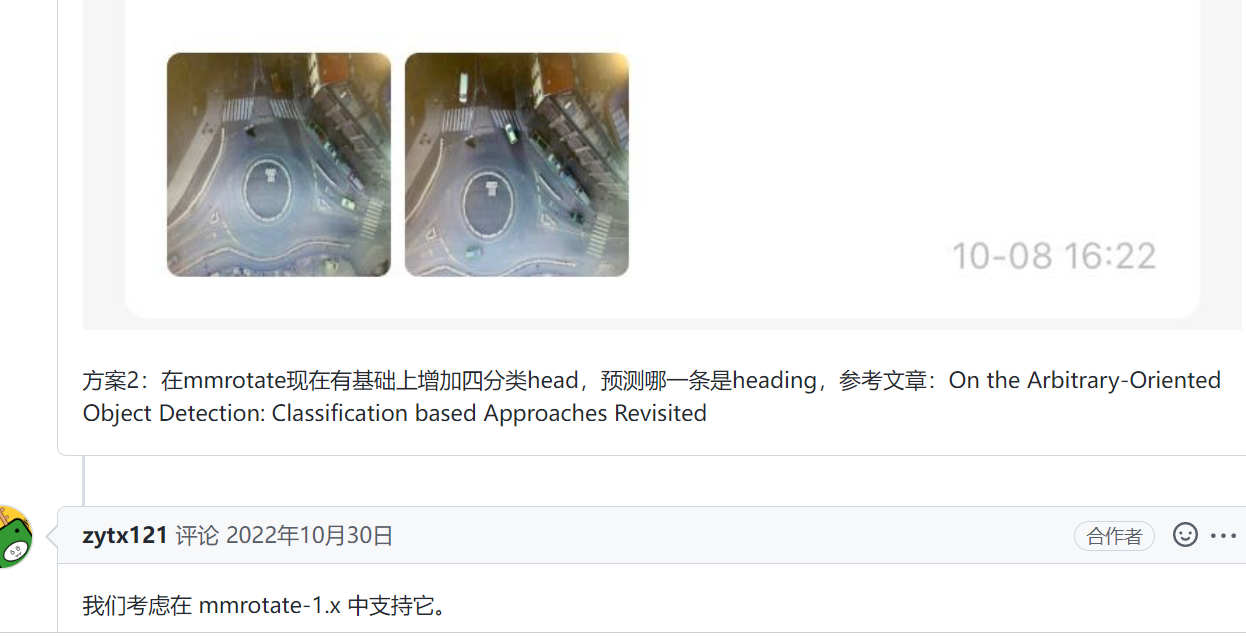
13、如何获得精度和F1分数
问题:

回答:
在我的例子中,我修改了 eval_map.py 和我的 custumdataset.py
通过在 def eval_rbbox_map 中创建额外的变量来计算
cls_all_tp = np.sum(tp) cls_all_fp = np.sum(fp)
参考我项目的代码
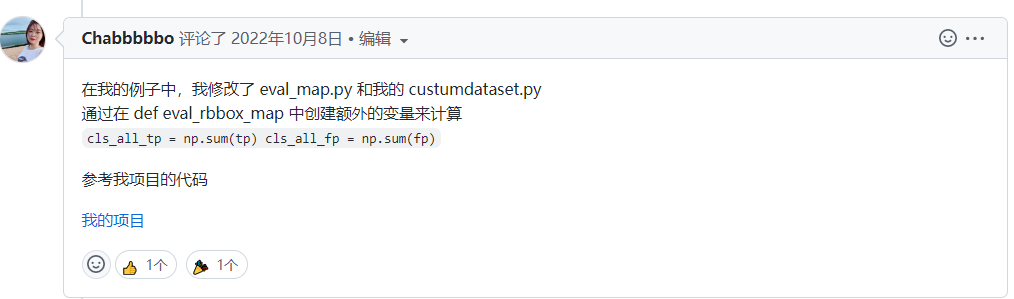
14、关于 R3Det 中的随机种子 #464
问题:
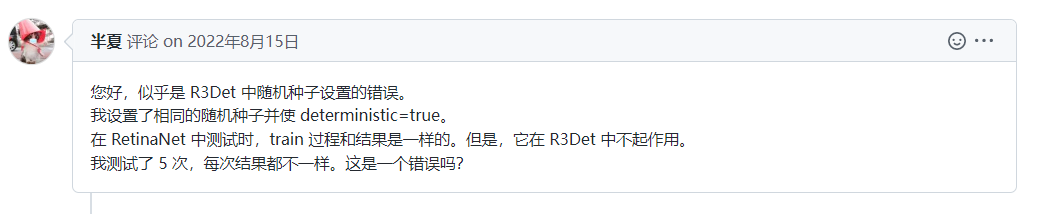

回答:

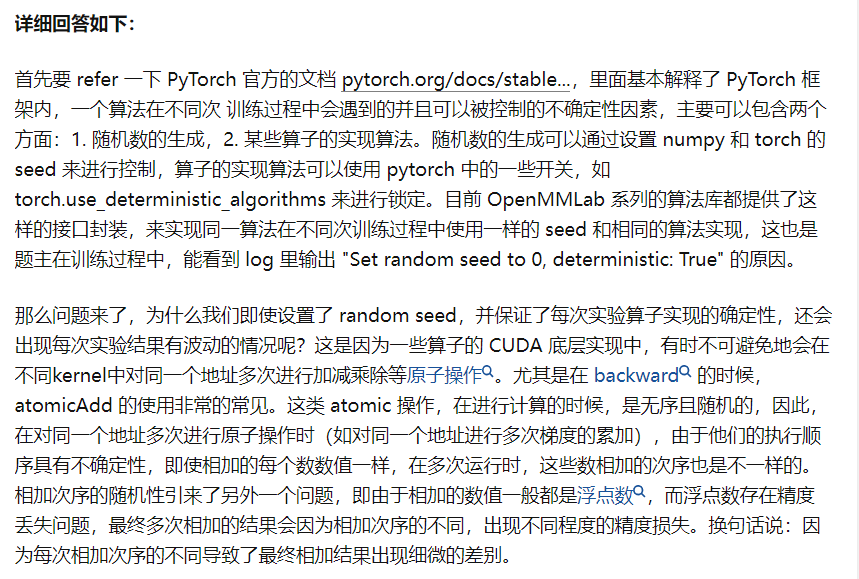
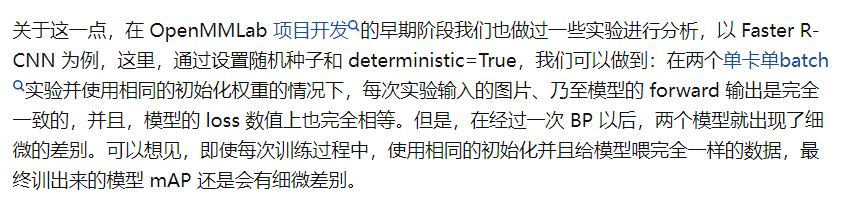
15、Question about random seed. #291
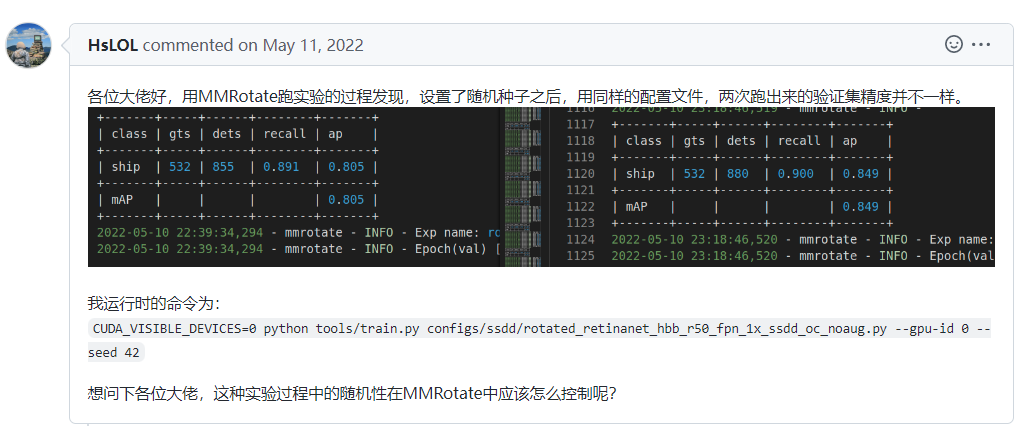
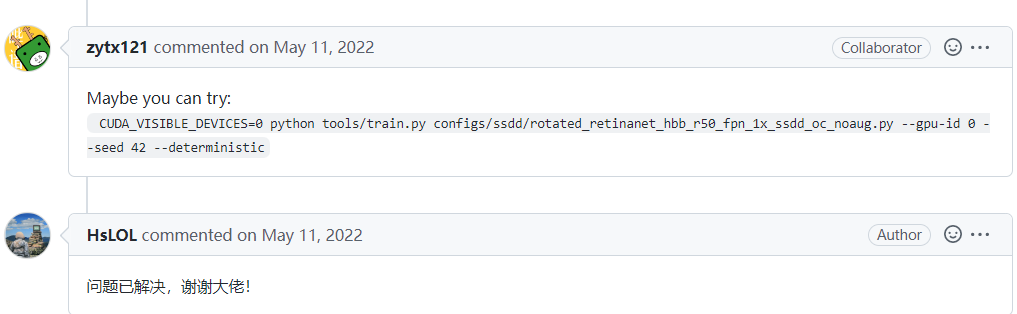
16、HRSC2016 数据集性能重新实现 #202
问题:
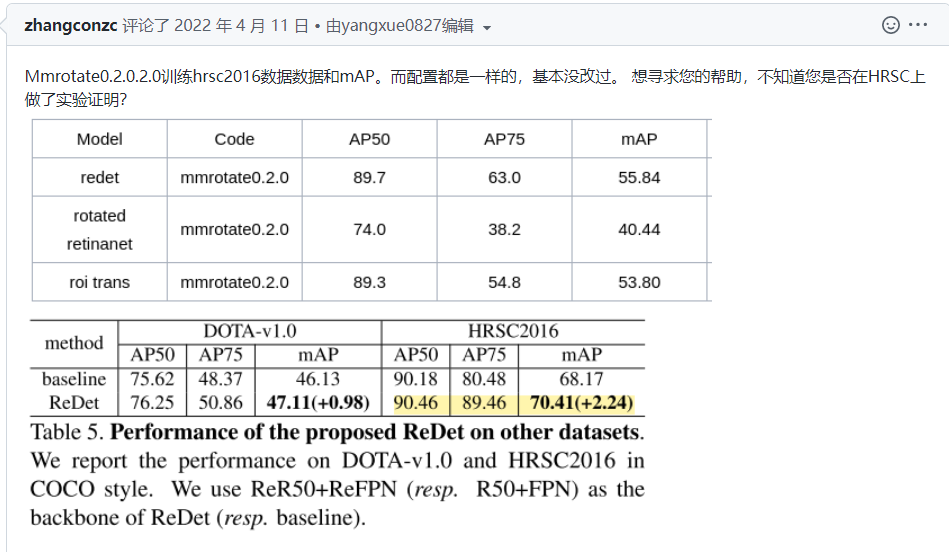
回答:
_base_ = [
'../_base_/datasets/hrsc.py', '../_base_/schedules/schedule_3x.py',
'../_base_/default_runtime.py'
]
angle_version = 'le90'
model = dict(
type='ReDet',
backbone=dict(
type='ReResNet',
depth=50,
num_stages=4,
out_indices=(0, 1, 2, 3),
frozen_stages=1,
style='pytorch',
pretrained='./work_dirs/re_resnet50_c8_batch256-25b16846.pth'),
neck=dict(
type='ReFPN',
in_channels=[256, 512, 1024, 2048],
out_channels=256,
num_outs=5),
rpn_head=dict(
type='RotatedRPNHead',
in_channels=256,
feat_channels=256,
version=angle_version,
anchor_generator=dict(
type='AnchorGenerator',
scales=[8],
ratios=[0.5, 1.0, 2.0],
strides=[4, 8, 16, 32, 64]),
bbox_coder=dict(
type='DeltaXYWHBBoxCoder',
target_means=[.0, .0, .0, .0],
target_stds=[1.0, 1.0, 1.0, 1.0]),
loss_cls=dict(
type='CrossEntropyLoss', use_sigmoid=True, loss_weight=1.0),
loss_bbox=dict(type='SmoothL1Loss', beta=1.0 / 9.0, loss_weight=1.0)),
roi_head=dict(
type='RoITransRoIHead',
version=angle_version,
num_stages=2,
stage_loss_weights=[1, 1],
bbox_roi_extractor=[
dict(
type='SingleRoIExtractor',
roi_layer=dict(
type='RoIAlign', output_size=7, sampling_ratio=0),
out_channels=256,
featmap_strides=[4, 8, 16, 32]),
dict(
type='RotatedSingleRoIExtractor',
roi_layer=dict(
type='RiRoIAlignRotated',
out_size=7,
num_samples=2,
num_orientations=8,
clockwise=True),
out_channels=256,
featmap_strides=[4, 8, 16, 32]),
],
bbox_head=[
dict(
type='RotatedShared2FCBBoxHead',
in_channels=256,
fc_out_channels=1024,
roi_feat_size=7,
num_classes=1,
bbox_coder=dict(
type='DeltaXYWHAHBBoxCoder',
angle_range=angle_version,
norm_factor=2,
edge_swap=True,
target_means=[0., 0., 0., 0., 0.],
target_stds=[0.1, 0.1, 0.2, 0.2, 0.1]),
reg_class_agnostic=True,
loss_cls=dict(
type='CrossEntropyLoss',
use_sigmoid=False,
loss_weight=1.0),
loss_bbox=dict(type='SmoothL1Loss', beta=1.0,
loss_weight=1.0)),
dict(
type='RotatedShared2FCBBoxHead',
in_channels=256,
fc_out_channels=1024,
roi_feat_size=7,
num_classes=1,
bbox_coder=dict(
type='DeltaXYWHAOBBoxCoder',
angle_range=angle_version,
norm_factor=None,
edge_swap=True,
proj_xy=True,
target_means=[0., 0., 0., 0., 0.],
target_stds=[0.05, 0.05, 0.1, 0.1, 0.05]),
reg_class_agnostic=False,
loss_cls=dict(
type='CrossEntropyLoss',
use_sigmoid=False,
loss_weight=1.0),
loss_bbox=dict(type='SmoothL1Loss', beta=1.0, loss_weight=1.0))
]),
train_cfg=dict(
rpn=dict(
assigner=dict(
type='MaxIoUAssigner',
pos_iou_thr=0.7,
neg_iou_thr=0.3,
min_pos_iou=0.3,
match_low_quality=True,
ignore_iof_thr=-1),
sampler=dict(
type='RandomSampler',
num=256,
pos_fraction=0.5,
neg_pos_ub=-1,
add_gt_as_proposals=False),
allowed_border=0,
pos_weight=-1,
debug=False),
rpn_proposal=dict(
nms_pre=2000,
max_per_img=2000,
nms=dict(type='nms', iou_threshold=0.7),
min_bbox_size=0),
rcnn=[
dict(
assigner=dict(
type='MaxIoUAssigner',
pos_iou_thr=0.5,
neg_iou_thr=0.5,
min_pos_iou=0.5,
match_low_quality=False,
ignore_iof_thr=-1,
iou_calculator=dict(type='BboxOverlaps2D')),
sampler=dict(
type='RandomSampler',
num=512,
pos_fraction=0.25,
neg_pos_ub=-1,
add_gt_as_proposals=True),
pos_weight=-1,
debug=False),
dict(
assigner=dict(
type='MaxIoUAssigner',
pos_iou_thr=0.5,
neg_iou_thr=0.5,
min_pos_iou=0.5,
match_low_quality=False,
ignore_iof_thr=-1,
iou_calculator=dict(type='RBboxOverlaps2D')),
sampler=dict(
type='RRandomSampler',
num=512,
pos_fraction=0.25,
neg_pos_ub=-1,
add_gt_as_proposals=True),
pos_weight=-1,
debug=False)
]),
test_cfg=dict(
rpn=dict(
nms_pre=2000,
max_per_img=2000,
nms=dict(type='nms', iou_threshold=0.7),
min_bbox_size=0),
rcnn=dict(
nms_pre=2000,
min_bbox_size=0,
score_thr=0.05,
nms=dict(iou_thr=0.1),
max_per_img=2000)))
img_norm_cfg = dict(
mean=[123.675, 116.28, 103.53], std=[58.395, 57.12, 57.375], to_rgb=True)
train_pipeline = [
dict(type='LoadImageFromFile'),
dict(type='LoadAnnotations', with_bbox=True),
dict(type='RResize', img_scale=(800, 512)),
dict(type='RRandomFlip', flip_ratio=0.5),
dict(type='Normalize', **img_norm_cfg),
dict(type='Pad', size_divisor=32),
dict(type='DefaultFormatBundle'),
dict(type='Collect', keys=['img', 'gt_bboxes', 'gt_labels'])
]
test_pipeline = [
dict(type='LoadImageFromFile'),
dict(
type='MultiScaleFlipAug',
img_scale=(800, 512),
flip=False,
transforms=[
dict(type='RResize'),
dict(type='Normalize', **img_norm_cfg),
dict(type='Pad', size_divisor=32),
dict(type='DefaultFormatBundle'),
dict(type='Collect', keys=['img'])
])
]
dataset_type = 'HRSCDataset'
data_root = '/data/dataset_share/HRSC2016/HRSC2016/'
data = dict(
samples_per_gpu=2,
workers_per_gpu=2,
train=dict(
type=dataset_type,
classwise=False,
ann_file=data_root + 'ImageSets/trainval.txt',
ann_subdir=data_root + 'FullDataSet/Annotations/',
img_subdir=data_root + 'FullDataSet/AllImages/',
pipeline=train_pipeline),
val=dict(
type=dataset_type,
classwise=False,
ann_file=data_root + 'ImageSets/test.txt',
ann_subdir=data_root + 'FullDataSet/Annotations/',
img_subdir=data_root + 'FullDataSet/AllImages/',
pipeline=test_pipeline),
test=dict(
type=dataset_type,
classwise=False,
ann_file=data_root + 'ImageSets/test.txt',
ann_subdir=data_root + 'FullDataSet/Annotations/',
img_subdir=data_root + 'FullDataSet/AllImages/',
pipeline=test_pipeline))
evaluation = dict(interval=12, metric='mAP')
optimizer = dict(lr=0.01)
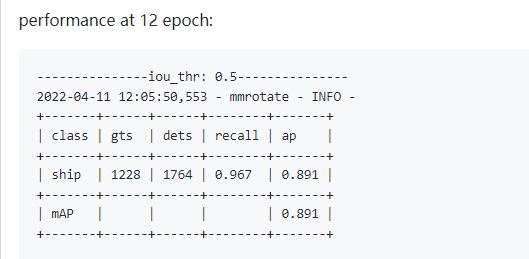
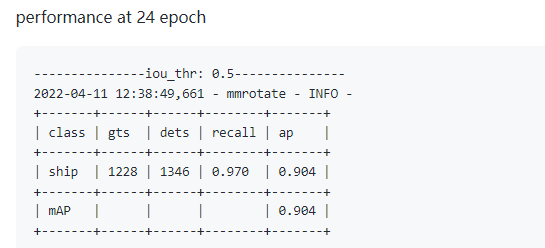
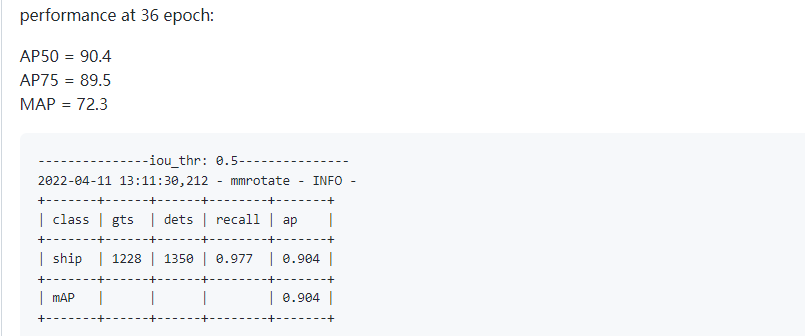
#原因是问题者的Target_stds和官方不一致,同时学习率也不一致导致的,官方也是使用单张GPU进行模型训练的。
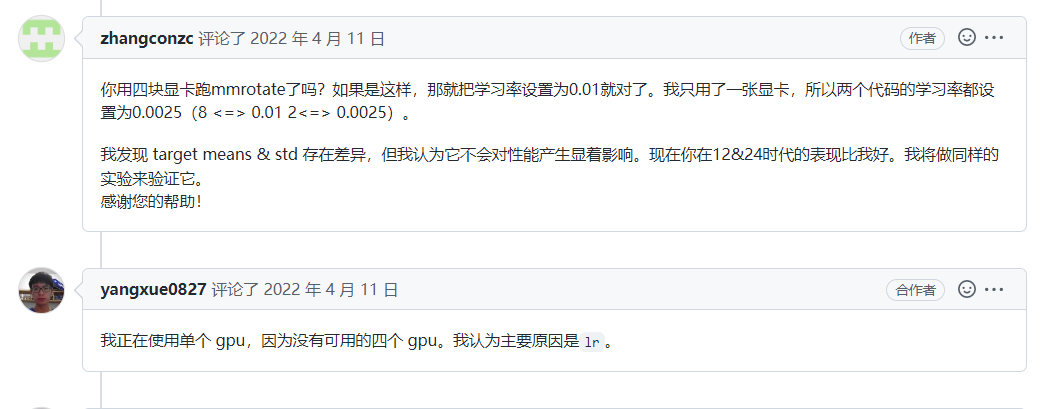
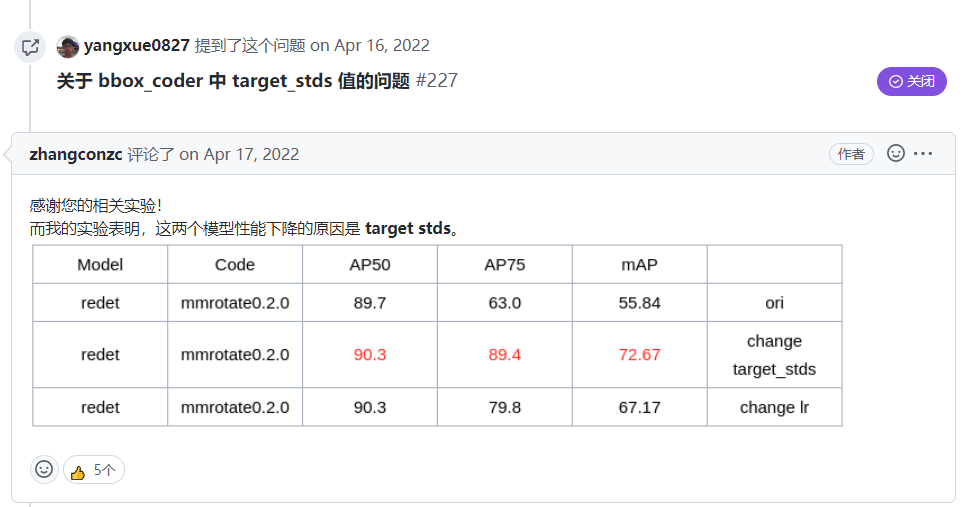
问题:
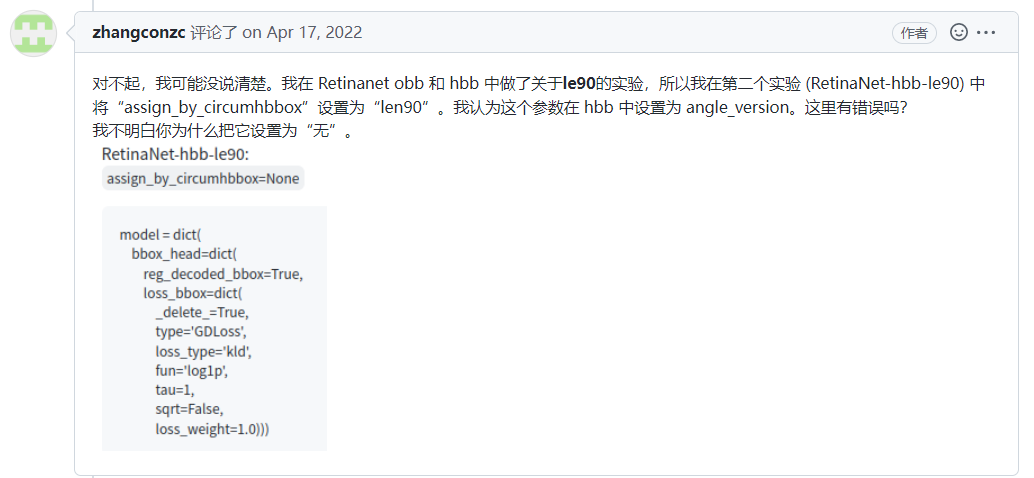
回答:
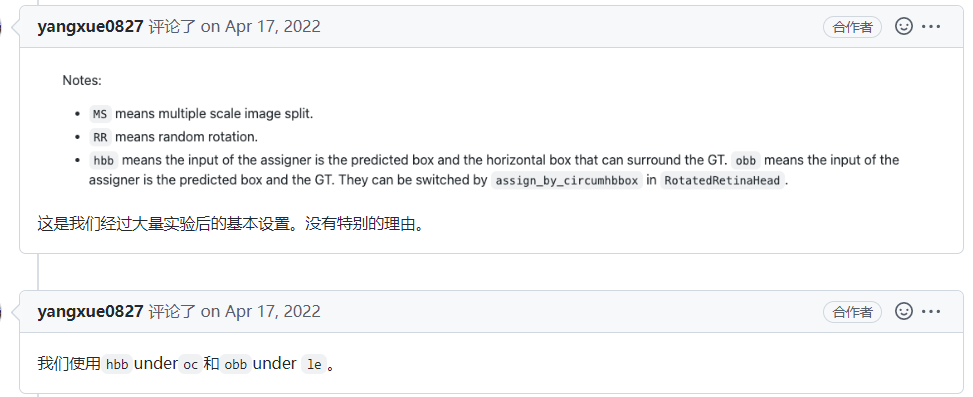
17、单个类别训练报错
问题:
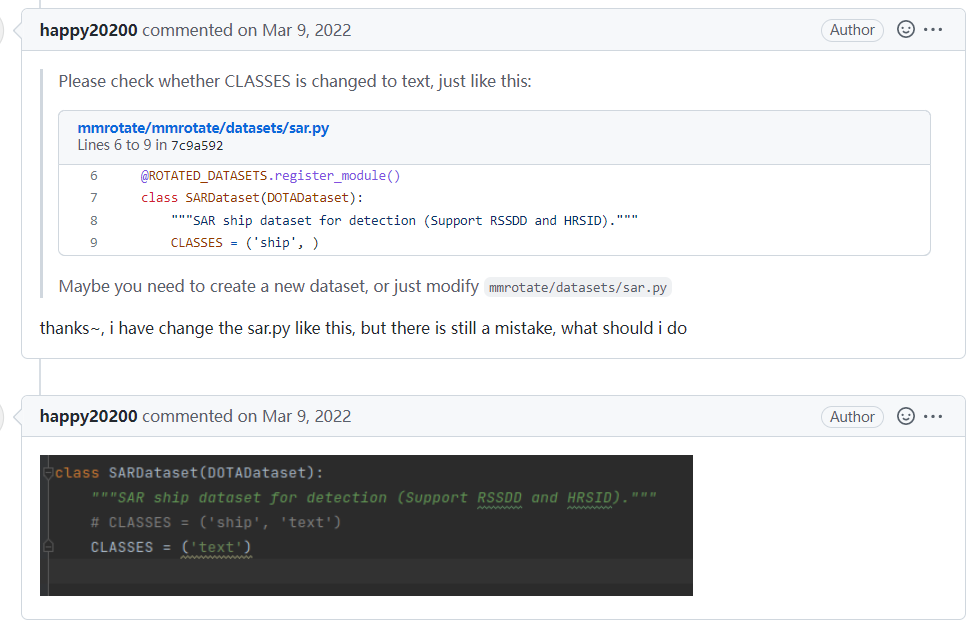
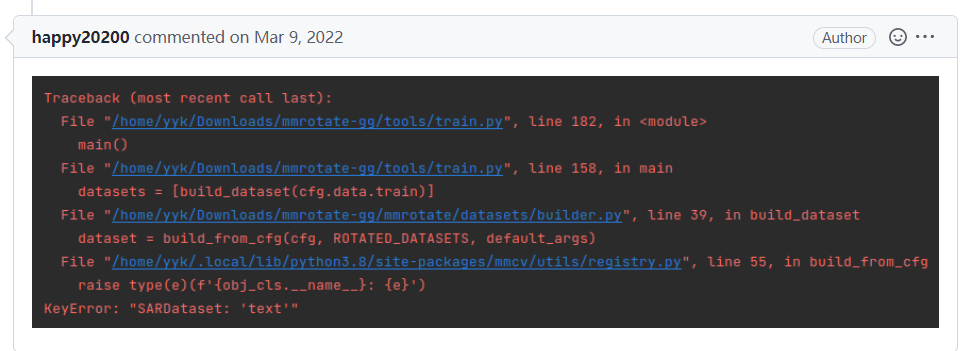
回答:

18、HRSC2016 的 classwise 设置为 True 时,在评估时出现“IndexError: tuple index out of range”。 #182
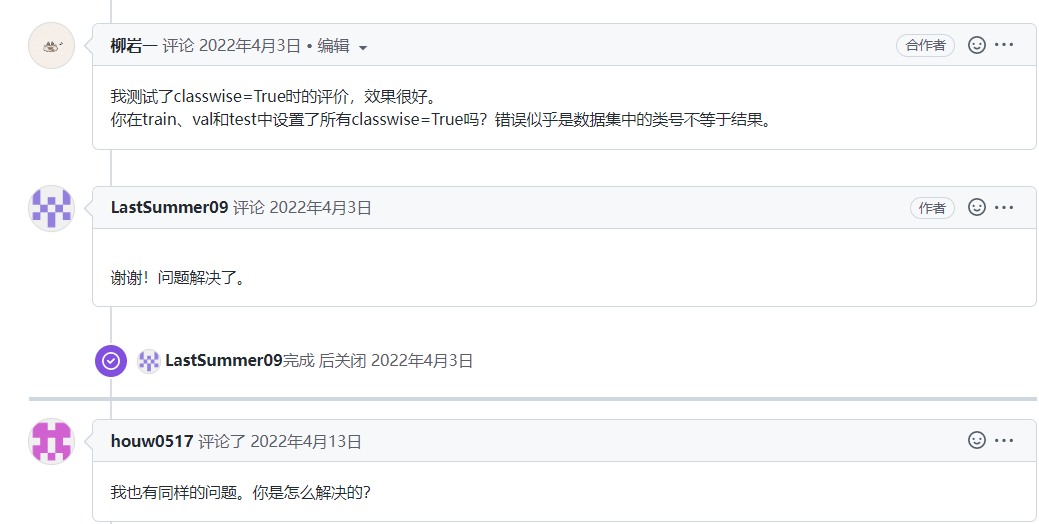
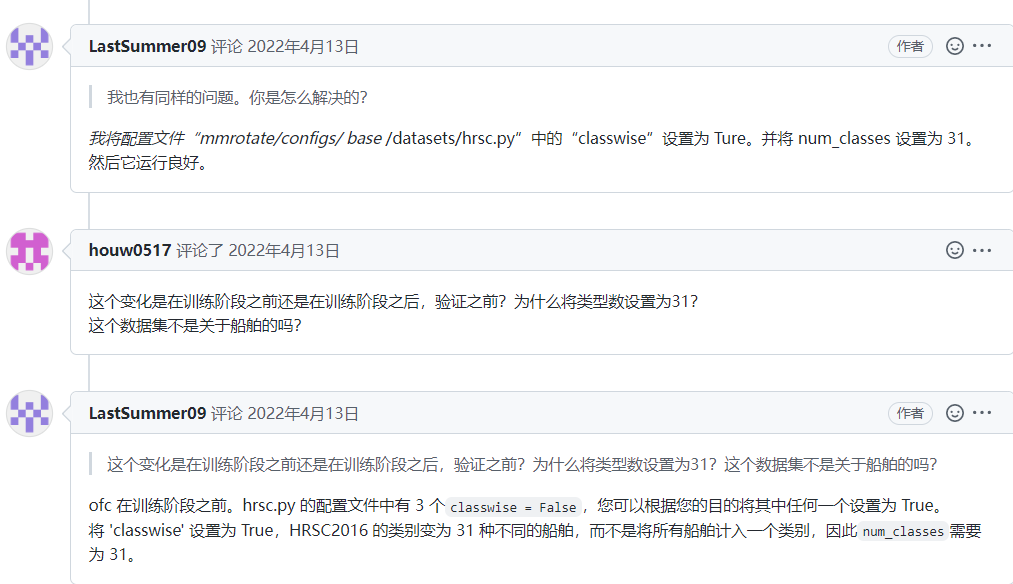
19、尝试结合 swin-Transform 和 s2anet #217
问题:

_base_ = ['./s2anet_r50_fpn_1x_dota_le135.py']
pretrained = 'https://github.com/SwinTransformer/storage/releases/download/v1.0.0/swin_tiny_patch4_window7_224.pth' # noqa
model = dict(
backbone=dict(
_delete_=True,
type='SwinTransformer',
embed_dims=96,
depths=[2, 2, 6, 2],
num_heads=[3, 6, 12, 24],
window_size=7,
mlp_ratio=4,
qkv_bias=True,
qk_scale=None,
drop_rate=0.,
attn_drop_rate=0.,
drop_path_rate=0.2,
patch_norm=True,
out_indices=(0, 1, 2, 3),
with_cp=False,
convert_weights=True,
init_cfg=dict(type='Pretrained', checkpoint=pretrained)),
neck=dict(
_delete_=True,
type='FPN',
in_channels=[96, 192, 384, 768],
out_channels=256,
num_outs=5))
optimizer = dict(
_delete_=True,
type='AdamW',
lr=0.0001,
betas=(0.9, 0.999),
weight_decay=0.05,
paramwise_cfg=dict(
custom_keys={
'absolute_pos_embed': dict(decay_mult=0.),
'relative_position_bias_table': dict(decay_mult=0.),
'norm': dict(decay_mult=0.)
}))
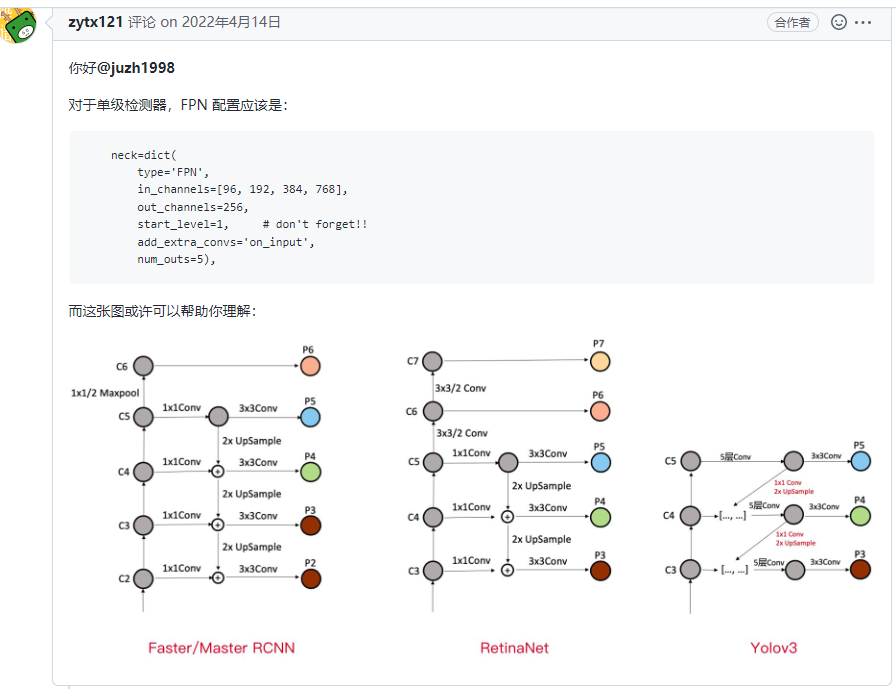
20、数据标签过多次显示会爆炸、预测后不出指标结果;当数据标签过多时,显存会爆,预测后指标结果不显示; #333



21、一个对象在 oriented-reppoints 中有两个预测类 #426
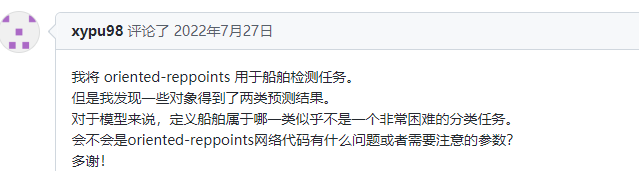
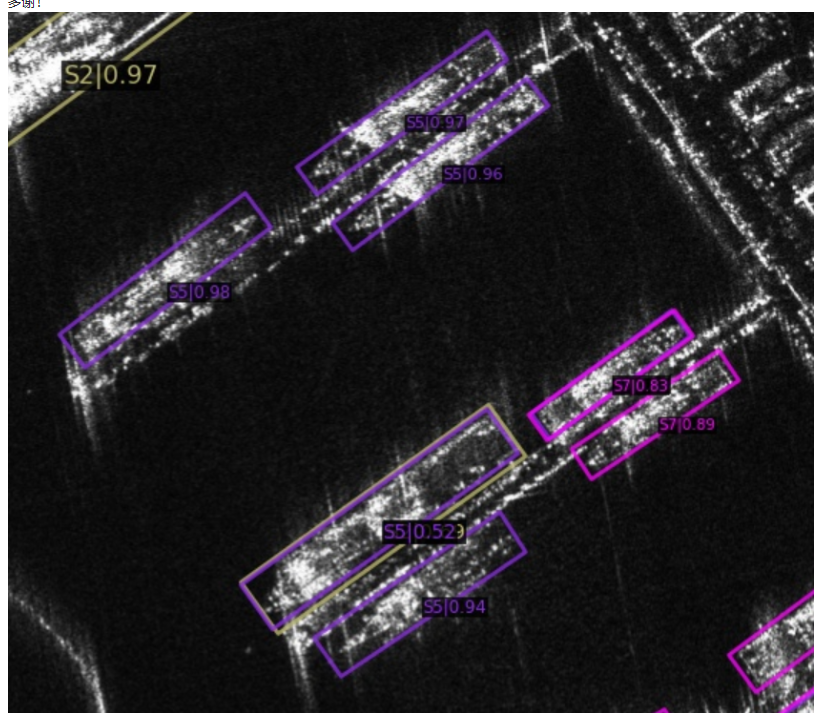
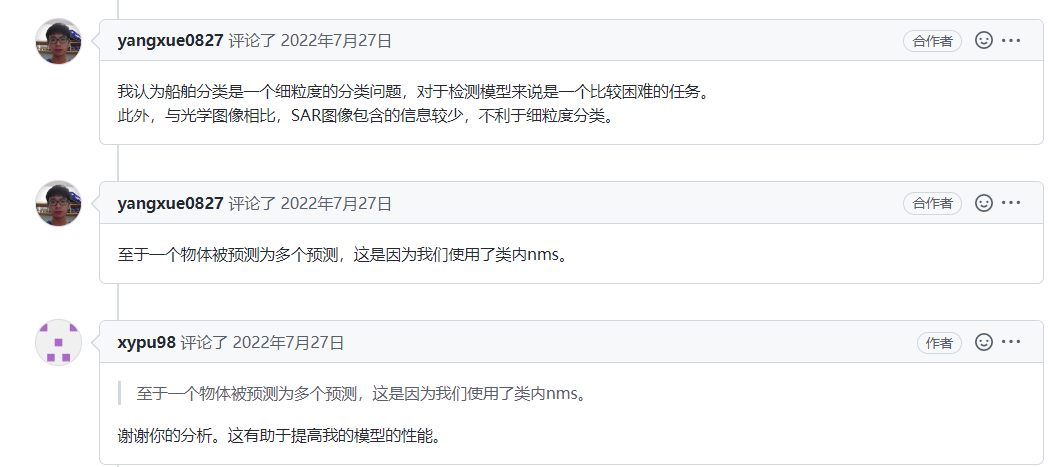
22、loss降不下来 #330
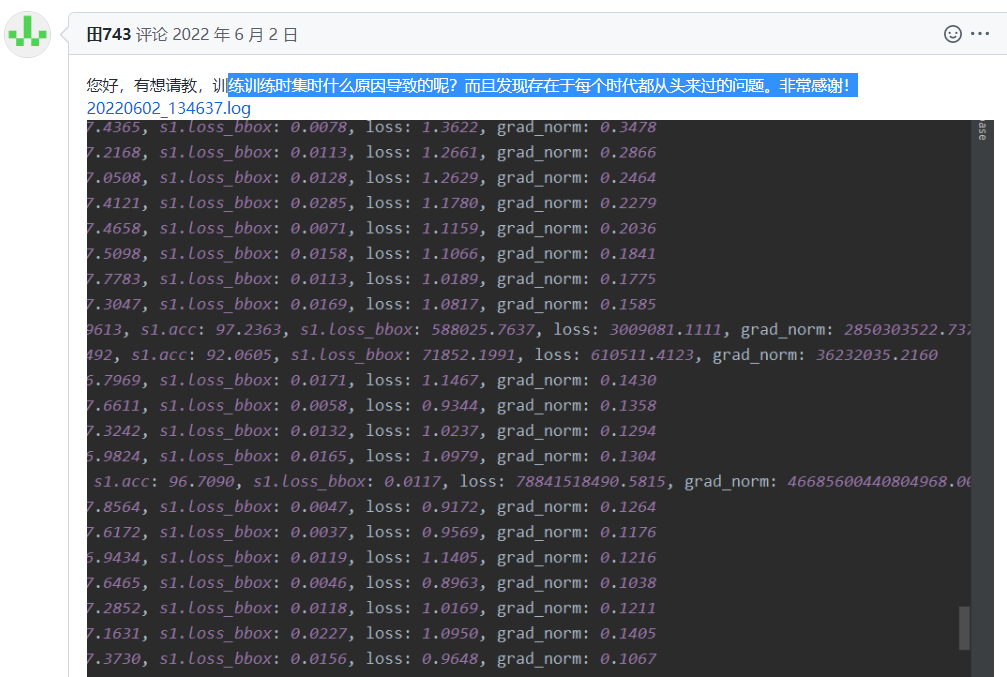

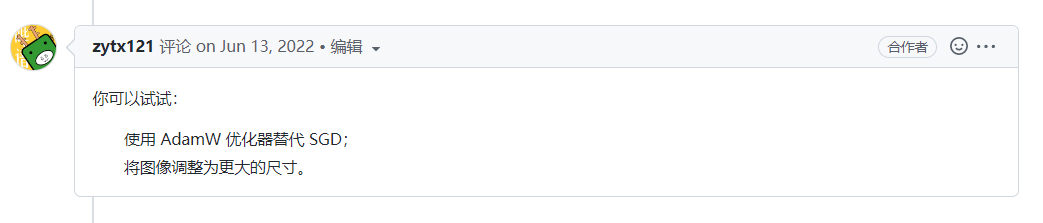
23、当我使用 rmosaic 时如何可视化 #686
你好@QAQTATQAQTAT,您可以使用demo/image_demo.py可视化 rmosaic 的输出。rmosaic的使用方法可以参考https://github.com/open-mmlab/mmrotate/blob/dev-1.x/configs/rotated_rtmdet/rotated_rtmdet_tiny-300e-aug-hrsc.py 。
Mosaic( img_scale=(1024, 1024))-> Resize(scale=(2048, 2048))->RandomCrop(crop_size=(1024, 1024))
train_pipeline = [
dict(
type='mmdet.LoadImageFromFile',
file_client_args={{_base_.file_client_args}}),
dict(type='mmdet.LoadAnnotations', with_bbox=True, box_type='qbox'),
dict(type='ConvertBoxType', box_type_mapping=dict(gt_bboxes='rbox')),
dict(
type='mmdet.CachedMosaic',
img_scale=(800, 800),
pad_val=114.0,
max_cached_images=20,
random_pop=False),
dict(
type='mmdet.RandomResize',
resize_type='mmdet.Resize',
scale=(1600, 1600),
ratio_range=(0.5, 2.0),
keep_ratio=True),
dict(type='RandomRotate', prob=0.5, angle_range=180),
dict(type='mmdet.RandomCrop', crop_size=(800, 800)),
dict(type='mmdet.YOLOXHSVRandomAug'),
dict(
type='mmdet.RandomFlip',
prob=0.75,
direction=['horizontal', 'vertical', 'diagonal']),
dict(type='mmdet.Pad', size=(800, 800), pad_val=dict(img=(114, 114, 114))),
dict(
type='mmdet.CachedMixUp',
img_scale=(800, 800),
ratio_range=(1.0, 1.0),
max_cached_images=10,
random_pop=False,
pad_val=(114, 114, 114),
prob=0.5),
dict(type='mmdet.PackDetInputs')
]
24、[WIP] 在 TRR360D 中支持 RR360(旋转矩形 360)检测 #731
https://github.com/open-mmlab/mmrotate/pull/731
25、Oriented RCNN 不支持 iou loss? #649

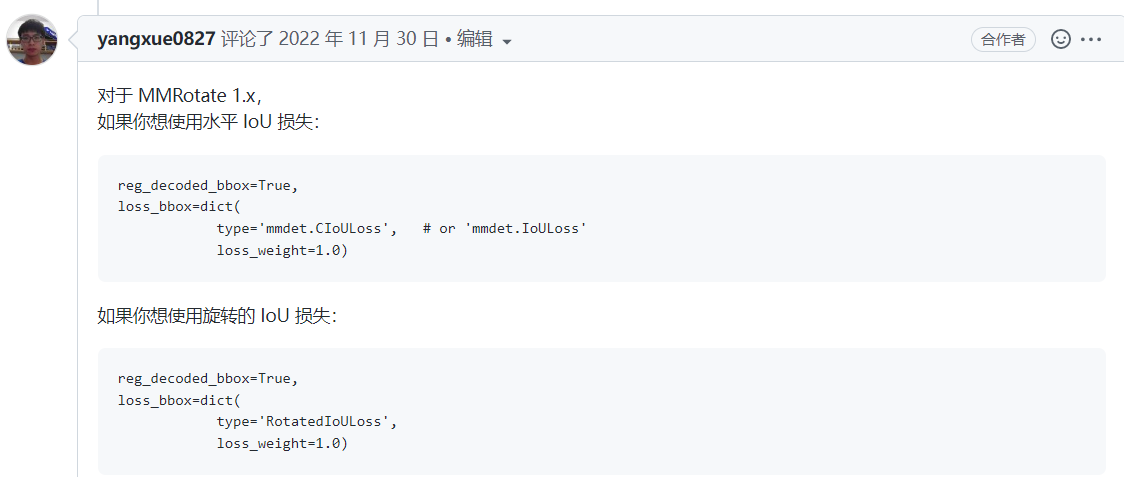
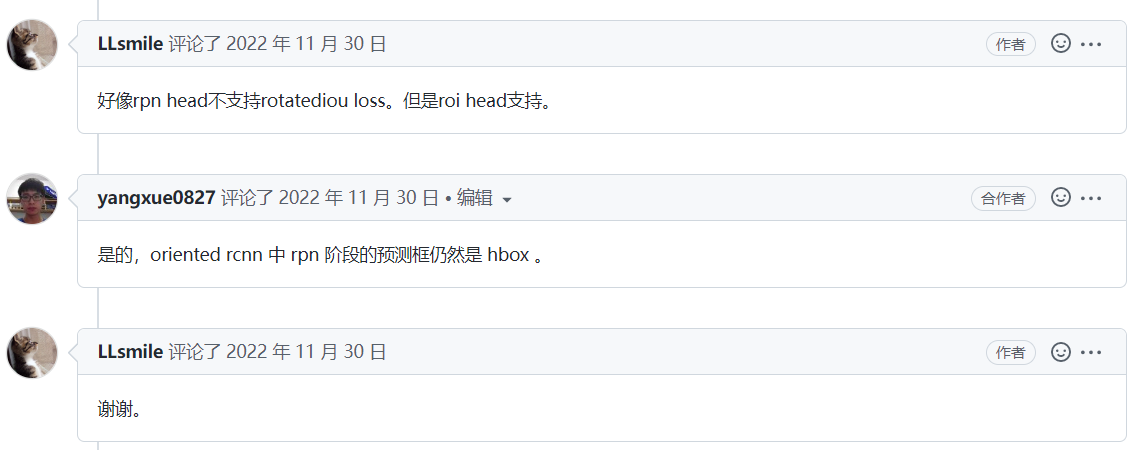